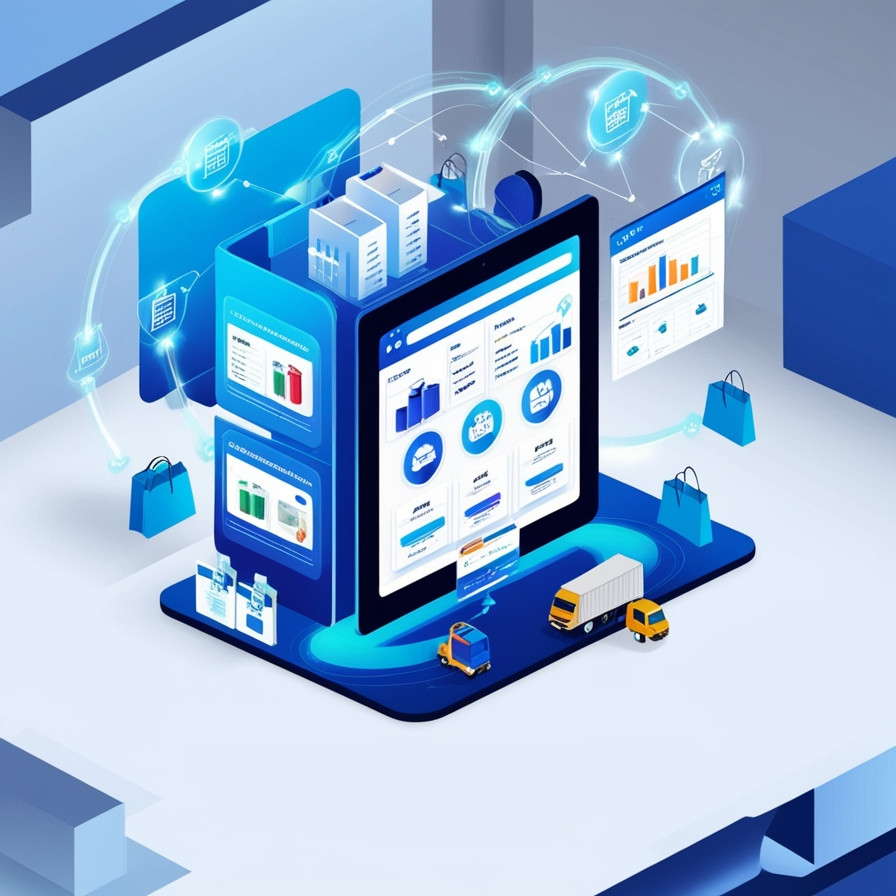
Predictive analytics provides a transformative solution, equipping businesses with the tools to forecast seasonal sales trends and turn data into actionable insights that drive profitability.
This article explores how predictive analytics can revolutionize seasonal sales trend prediction in retail, solving common business challenges and delivering actionable benefits for decision-makers.
The Challenges Facing Retailers Today
Retailers, especially those managing both brick-and-mortar stores and e-commerce platforms, face a trifecta of challenges:
1. Real-Time Analysis of Customer Data
Consumer preferences are constantly shifting, influenced by weather, social media trends, and global events. Traditional approaches to analyzing customer data struggle to keep pace with these rapid changes, resulting in missed opportunities for personalized marketing.
2. Demand Forecasting and Inventory Management
Stocking the proper inventory at the right time is critical. Overestimating demand leads to excess inventory and markdowns, while underestimating demand results in stockouts and missed sales. Achieving this balance is particularly tricky during seasonal peaks.
3. Data Silos Across Channels
Retailers often struggle with fragmented data systems. Physical stores and online platforms generate disparate data sets, making it difficult to gain a unified view of customer behavior and inventory performance.
Without addressing these challenges, retailers risk losing their competitive edge in a saturated market.
The Role of Predictive Analytics in Addressing These Challenges
Predictive analytics—powered by advanced algorithms and artificial intelligence—has emerged as a cornerstone for modern retail strategies. Predictive models can accurately forecast future sales patterns by analyzing historical data, current trends, and external factors.
Here’s how predictive analytics tackles the key challenges:
1. Personalized Marketing Through Real-Time Insights
Predictive analytics enables retailers to analyze customer data in real-time, identifying patterns and preferences that inform hyper-personalized marketing strategies. For instance, AI-driven algorithms can segment customers based on their purchasing history and browsing behavior, tailoring promotions to individual needs.
Example: A retailer uses predictive analytics to identify customers likely to shop for winter wear in October. The retailer boosts early-season sales by launching targeted email campaigns with curated recommendations.
2. Optimizing Inventory Based on Demand Forecasting
By leveraging predictive analytics, retailers can anticipate which products will sell during specific seasons and adjust inventory levels accordingly. These insights help reduce overstock and minimize stockouts.
Example: A retailer forecasts a spring demand surge for floral dresses. With this knowledge, they optimize inventory and avoid losing sales due to shortages or markdowns on unsold stock.
3. Unifying Data Across Omnichannel Platforms
Predictive analytics platforms integrate data from physical stores, e-commerce websites, and social media channels. This centralized approach provides a 360-degree view of customer behavior and inventory performance, empowering retailers to make informed decisions.
Example: A retailer tracks a customer’s in-store purchase history and online browsing patterns. Predictive analytics highlights that the customer frequently buys accessories with their apparel, prompting the retailer to bundle products and increase cart value.
The Benefits of Predicting Seasonal Sales Trends
Retailers who adopt predictive analytics for seasonal sales trend forecasting can unlock a myriad of benefits:
1. Increased Sales and Revenue
Accurate forecasts enable retailers to align inventory with demand, reducing lost sales opportunities and maximizing revenue during peak seasons.
2. Improved Customer Experience
By anticipating customer needs, retailers can deliver personalized recommendations, faster deliveries, and seamless shopping experiences, building stronger brand loyalty.
3. Reduced Costs
Optimized inventory levels mean fewer markdowns on unsold products and lower carrying costs, significantly improving profit margins.
4. Streamlined Supply Chain Operations
Predictive analytics helps retailers plan their logistics more effectively, ensuring that the right products reach the right locations at the right time.
5. Data-Driven Decision-Making
Centralized and integrated data systems empower decision-makers with actionable insights, reducing guesswork and enabling proactive strategies.
Real-World Applications: Retail Success Stories
To illustrate the transformative impact of predictive analytics, let’s look at real-world applications:
Case Study 1: Enhancing Seasonal Promotions A global retailer leveraged predictive analytics to analyze historical sales data and external factors like weather forecasts. The insights revealed that demand for outerwear surged during unexpected cold snaps. Armed with this knowledge, the retailer executed location-specific promotions, driving a 20% increase in seasonal sales.
Case Study 2: Reducing Waste Through Inventory Optimization An e-commerce brand used predictive analytics to forecast demand for trending items during the holiday season. The model’s accuracy led to a 30% reduction in overstocked inventory and a 15% decrease in lost sales due to stockouts.
Case Study 3: Unifying Data for Omnichannel Success A retail chain integrated its physical and online data using a predictive analytics platform. This unified approach enabled the brand to identify high-performing products across channels, enhancing cross-selling opportunities and improving overall sales by 25%.
Implementing Predictive Analytics: Actionable Steps for Retailers
Transitioning to a predictive analytics-driven approach doesn’t have to be daunting. Here’s a roadmap for retailers looking to harness the power of predictive analytics:
Step 1: Invest in the Right Technology
Choose predictive analytics platforms that align with your business needs, offering real-time data integration and AI-driven forecasting capabilities.
Step 2: Integrate Data Systems
Break down silos by centralizing data from all channels, including physical stores, e-commerce sites, and external sources like social media and weather forecasts.
Step 3: Collaborate Across Teams
Ensure marketing, inventory management, and supply chain teams collaborate, leveraging predictive insights to align strategies.
Step 4: Start Small and Scale
Begin with a pilot project focused on a specific product line or season. Use the results to refine your approach before scaling to other areas.
Step 5: Monitor and Adapt
Continuously analyze the performance of your predictive models, making adjustments as needed to stay ahead of changing market dynamics.
The Future of Retail: Data-Driven Success
Adopting predictive analytics isn’t just a trend—it’s necessary for retailers aiming to thrive in today’s competitive landscape. By accurately predicting seasonal sales trends, retailers can unlock new levels of efficiency, enhance customer experiences, and drive significant revenue growth.
The key to success lies in embracing data-driven decision-making and fostering a culture of innovation within your organization.
Stay Ahead of the Curve
Are you ready to transform your retail business with predictive analytics? Don’t let outdated strategies hold you back from achieving your full potential. Contact us today to learn how our cutting-edge solutions can help you forecast seasonal trends, optimize inventory, and deliver personalized customer experiences that set you apart.