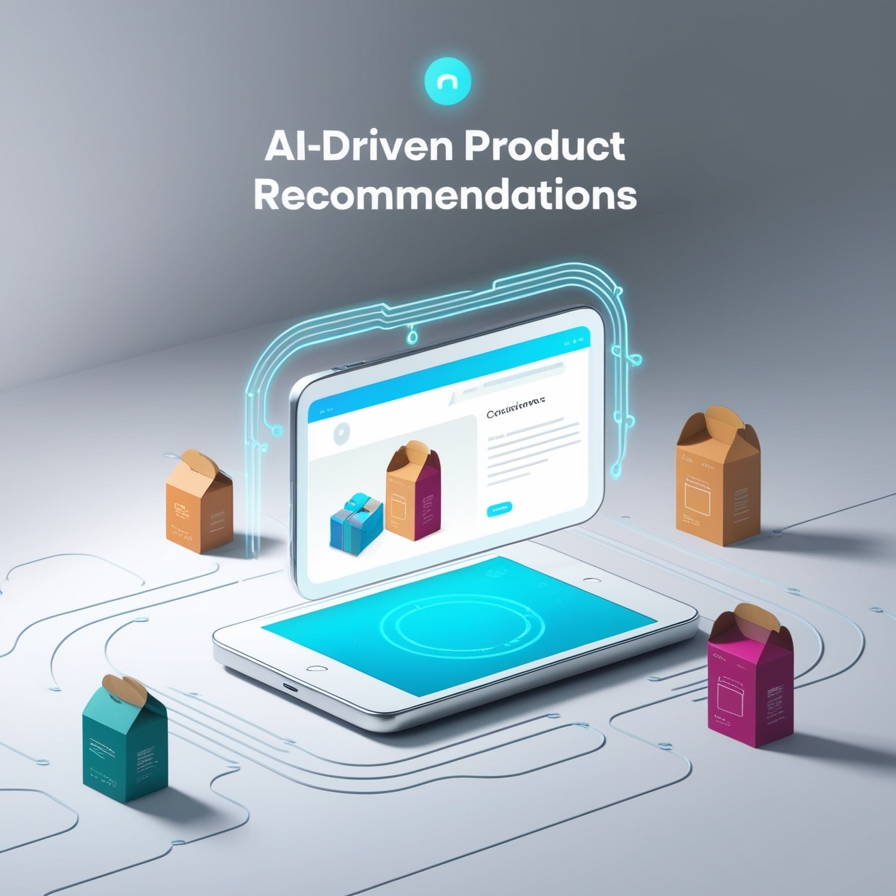
The e-commerce landscape has been revolutionized by the advent of artificial intelligence (AI), and one of the most impactful applications of AI in this space is improving product recommendations. As online shopping continues to grow, competition among retailers has intensified. Consumers now expect personalized, relevant, and efficient shopping experiences. E-commerce platforms increasingly leverage AI to provide better product recommendations to meet these expectations. This enhances customer satisfaction and drives higher sales and customer retention.
Understanding AI in Product Recommendations
AI-driven product recommendations are systems that leverage machine learning (ML), deep learning, and other AI technologies to analyze user data, identify patterns, and predict what products a customer might be interested in. These recommendations are typically based on factors like previous shopping behavior, search history, demographic data, and even real-time interaction on the website.
Traditional product recommendation systems in e-commerce relied heavily on collaborative and content-based filtering. While these techniques are still helpful, AI has introduced new levels of sophistication, enabling more accurate and personalized recommendations. Machine learning algorithms can process vast amounts of data in real-time, learning from every customer interaction to continuously improve recommendations.
AI Technologies Powering Product Recommendations
Several AI technologies are at the heart of modern product recommendation systems. These include:
1. Machine Learning (ML)
ML algorithms can analyze large datasets, learning from customer behavior to predict which products will be of interest. ML models are trained using historical data, including user clicks, searches, purchases, and more. Over time, the system can recognize patterns and trends, which allow it to suggest products that align with a shopper's preferences. These models can improve automatically as they gather more data, creating a continuously evolving recommendation system.
2. Deep Learning
Deep learning models, particularly those based on neural networks, excel at capturing complex patterns in data. In the context of product recommendations, deep learning can help identify subtle relationships between products and users. For example, a deep learning model could recognize that customers who buy hiking boots often also buy outdoor jackets, even if these products aren't frequently bought together. The power of deep learning lies in its ability to process unstructured data, such as product descriptions, images, and even customer reviews, and make relevant recommendations.
3. Natural Language Processing (NLP)
NLP is a subset of AI that enables machines to understand and interpret human language. In e-commerce, NLP analyzes customer reviews, product descriptions, and search queries to recommend products. NLP-based systems can understand the intent behind a customer's query and suggest more relevant items than those identified by keyword matching alone. By understanding the nuances of language, NLP helps create a more human-like interaction between customers and e-commerce platforms.
4. Reinforcement Learning
Reinforcement learning is another AI technique used in product recommendations, where the system learns from real-time feedback. When a customer interacts with recommended products, the system adjusts future recommendations based on these interactions. For example, if a customer clicks on a recommended product but doesn't purchase it, the algorithm learns that this type of recommendation may not be effective. Over time, the system improves by continually refining its recommendations.
5. Computer Vision
Computer vision is crucial in visually driven products like fashion and home decor. AI can analyze product images and use image recognition to recommend visually similar items. For example, if a customer is browsing a blue denim jacket, the system can recommend other jackets with similar styles or colors. Computer vision enables e-commerce platforms to cater to the visual preferences of shoppers, improving the relevance of recommendations.
Benefits of AI in Product Recommendations
Integrating AI into product recommendation systems provides several significant benefits for both e-commerce retailers and consumers:
1. Personalization
One of AI's most compelling advantages is its ability to deliver highly personalized shopping experiences. AI-powered systems can consider a customer's past behavior, demographic information, browsing history, and real-time interactions. This level of personalization means customers are more likely to see products that match their tastes, which increases the chances of a purchase. Moreover, personalization enhances customer satisfaction, making shoppers feel understood and valued.
2. Improved Conversion Rates
AI-driven product recommendations can increase conversion rates by showing customers products they are more likely to buy. Customers are more likely to add these products to their carts when presented with items that align with their preferences. Additionally, AI can optimize the timing and placement of recommendations, ensuring that products are suggested immediately in the shopping journey. This reduces friction in the buying process and increases the likelihood of a sale.
3. Increased Average Order Value (AOV)
AI can also help increase the average order value by suggesting complementary products through techniques like cross-selling and upselling. For example, when a customer adds a smartphone to their cart, the system may recommend accessories such as a phone case, screen protector, or wireless earbuds. These personalized suggestions encourage customers to spend more, increasing revenue for e-commerce platforms.
4. Better Customer Retention
Practical product recommendations can improve customer retention by providing a better shopping experience. When customers find that an e-commerce platform consistently recommends products that meet their needs and preferences, they are more likely to return for future purchases. AI can also re-engage customers who haven't purchased in a while by recommending products based on their previous activity.
5. Enhanced Customer Experience
AI-driven product recommendations improve the overall customer experience by making the shopping process faster, more intuitive, and more enjoyable. Shoppers no longer have to spend time searching through endless product listings to find what they want. Instead, AI can give them tailored suggestions, reducing decision fatigue and improving customer satisfaction.
Challenges of Implementing AI in Product Recommendations
While AI offers numerous benefits for e-commerce product recommendations, there are also challenges that retailers must address:
1. Data Privacy Concerns
AI systems rely heavily on data to deliver personalized recommendations. However, this raises concerns about how customer data is collected, stored, and used. With increasing regulations around data privacy, such as the General Data Protection Regulation (GDPR) in Europe, e-commerce platforms must ensure they are transparent about how they use customer data and implement robust security measures to protect it.
2. Cold Start Problem
The cold start problem occurs when a new user or product is added to the system, and there is insufficient data to generate accurate recommendations. AI systems need historical data to make informed predictions, so they may struggle to recommend products to new users or suggest newly added items. Solving the cold start problem often requires hybrid approaches, combining collaborative filtering with content-based methods or leveraging demographic information to generate initial recommendations.
3. Algorithmic Bias
AI models can sometimes exhibit biases based on the data they are trained on. If historical data reflects biased patterns, such as gender or racial preferences, AI algorithms may inadvertently reinforce these biases in their recommendations. Retailers must be aware of this issue and actively work to mitigate bias by using diverse and representative datasets.
4. Technical Complexity
Implementing AI-driven recommendation systems can be technically complex and resource-intensive. It requires skilled data scientists, engineers, and a robust computational infrastructure. Smaller e-commerce businesses may face challenges in adopting these technologies due to the costs and expertise required.
Real-World Applications of AI in Product Recommendations
AI-powered product recommendations are already being used by e-commerce platforms with great success. Here are some examples:
1. Amazon
Amazon's recommendation engine is one of the most well-known and practical examples of AI in e-commerce. It uses collaborative filtering, content-based filtering, and deep learning to recommend products based on a user's browsing history, past purchases, and items in their cart. Amazon's AI algorithms constantly learn and refine recommendations, contributing significantly to the company's sales.
2. Netflix
While Netflix is a streaming service rather than an e-commerce platform, its recommendation algorithms provide a valuable case study. Netflix uses AI to recommend TV shows and movies to its users based on viewing history, ratings, and preferences. The company's sophisticated recommendation system helps improve user engagement and retention, demonstrating the power of AI in creating personalized experiences.
3. Shopify
Shopify, a popular e-commerce platform for small to medium-sized businesses, offers AI-powered product recommendations as part of its services. To suggest relevant products, Shopify's algorithms analyze customer behavior, such as browsing and purchase history. As a result, smaller retailers can offer personalized shopping experiences without having to develop their own recommendation engines.
Conclusion
AI has fundamentally transformed the way e-commerce platforms recommend products to their customers. By leveraging machine learning, deep learning, NLP, and other AI technologies, retailers can offer personalized, relevant, and timely product suggestions that improve customer satisfaction, boost sales, and increase retention.
However, implementing AI-powered product recommendations is not without its challenges. Data privacy, bias, and the cold start problem are all issues that must be addressed. Despite these challenges, the benefits of AI in enhancing product recommendations are undeniable. We can expect even more sophisticated and accurate recommendation systems as AI technologies evolve.
For e-commerce businesses, investing in AI-driven product recommendations is not just a competitive advantage -it's becoming necessary in today's fast-paced digital marketplace.